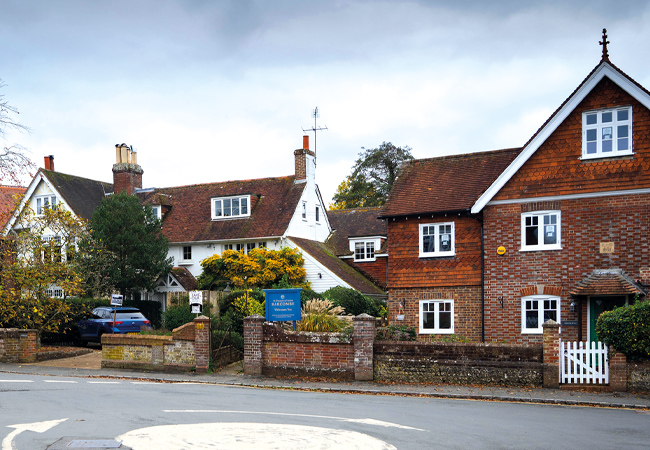
Barcombe village is not on the gas grid
Buro Happold is using the village of Barcombe, in East Sussex, to develop a roadmap to show how rural communities off the gas grid can switch to low carbon electric heating in a planned and affordable way.
The consultant is working with local community energy group Ovesco, electricity distributor UK Power Networks (UKPN), and Community Energy South, under the CommuniHeat partnership. The key output from this project will be a publication on how to approach decarbonising heating in off-grid rural communities across the UK.
Phil Proctor, associate director, Buro Happold Energy, says the biggest potential barrier to the decarbonisation of heat in Barcombe, as with most rural villages, is a lack of capacity in the electricity network. He sums the situation up succinctly: ‘Barcombe is a rural village of 700 homes; it is not on the gas grid, it burns oil for heating, locals travel higher-than-average miles, and it’s located on a weak part of the electricity network.’
Proctor says having UKPN on board is key to making the transition to low carbon heating affordable. ‘We know we’ll need to invest in the electricity network, but we need to do so in a planned way to minimise the number of interventions [and, hence, cost to consumers], rather than UKPN reacting on an ad hoc basis,’ he explains.
In Barcombe, the focus of decarbonising heat is on individual choice, but supported by access to community services, such as community assets that may include heat networks and renewable generation. A range of low carbon heating technologies is available, including low-temperature heat pumps, high-temperature heat pumps and direct electric boilers. Which goes into a home, and how it performs, will determine how much electricity is required and when. ‘In an unplanned approach, the network has no influence or sight of consumer choices – and yet it has to be able to react and respond to them,’ Proctor says.
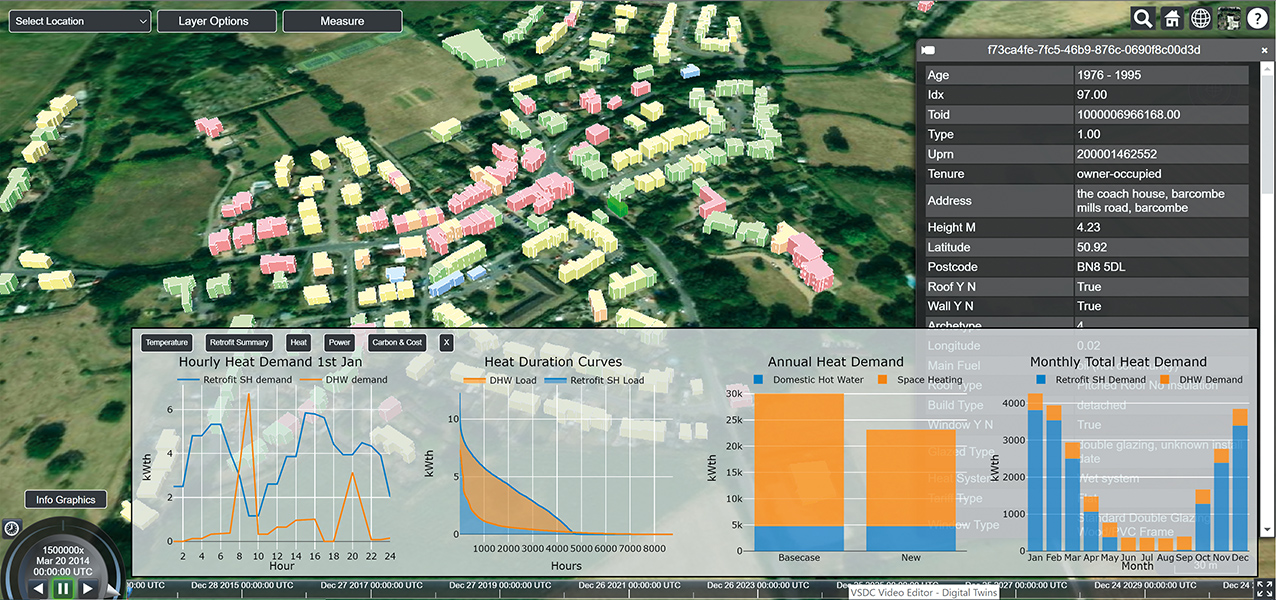
Screenshot of Barcombe's digital twin, with predicted heat demand. The coloured blocks represent different building typologies
To help establish the potential impacts of the different heating technologies and of additional energy efficiency measures, a digital twin of the village has been created (Figure 1). ‘You have to bring all the data into one environment to link it together; that way, you can collectively make the right decisions,’ explains Proctor.
Populating the model with the heat demand for each building has been a challenge. ‘Publicly available data is bad in terms of predicting what is needed; it has to be refined with data from the community, otherwise your predictions will be way out,’ says Proctor.
To establish the current heat demand, the village was divided into building archetypes. Publicly available data, such as Energy Performance Certificates (EPCs), was initially mapped onto each type. With help from Ovesco, surveys were then undertaken on a selection of archetypes, with the full support of community members. In a number of cases, data loggers were also installed into homes to get a more refined view of heat-consumption patterns. The information gathered has been revealing.
The survey data is showing that buildings are about 30% more efficient than EPC data is telling us. That has big implications, because you’ll be oversizing your networks and over estimating bills by a huge margin
‘The survey data is showing that buildings are about 30% more efficient than EPC data is telling us,’ says Proctor. ‘That has big implications, because you’ll be oversizing your networks and overestimating bills by a huge margin by relying on EPC data.’
Where a community heat network is proposed, the digital twin includes a script to optimise the network based on load diversity and heat-profile estimates. ‘If we wanted to put a heat network into a village, the model would tell us where to put it and which buildings to connect to it,’ Proctor explains.
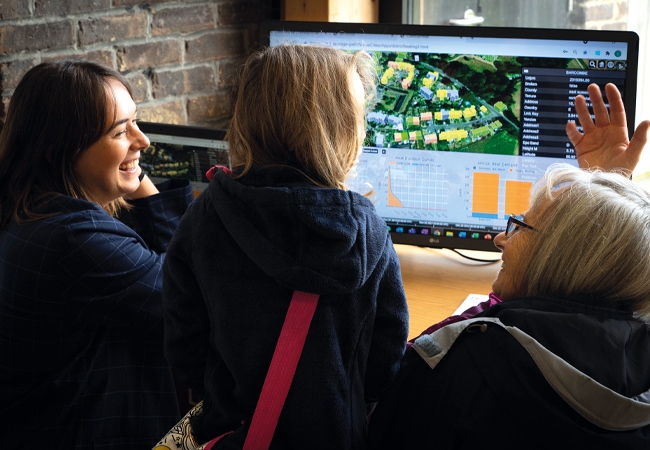
Community energy engagement using the digital twin
Alongside heat data, the model includes power network data. ‘A network diagram normally shows the meter and not the property behind the meter; we’re adding intelligence the other side of the meter, to inform the network strategy,’ Proctor adds. An inbuilt engine sizes the power network based on loads, and geospatially aligns the network data with building data. The modelling represents the first step in what is a multi-step process of modelling, developing an implementation road map, and designing the solution, which can all be done in the same digital environment.
Having populated the model, Buro Happold is now starting to model various scenarios. ‘It’s scenario-based, because we do not currently know what technologies consumers will choose,’ says Proctor (see Figure 2).
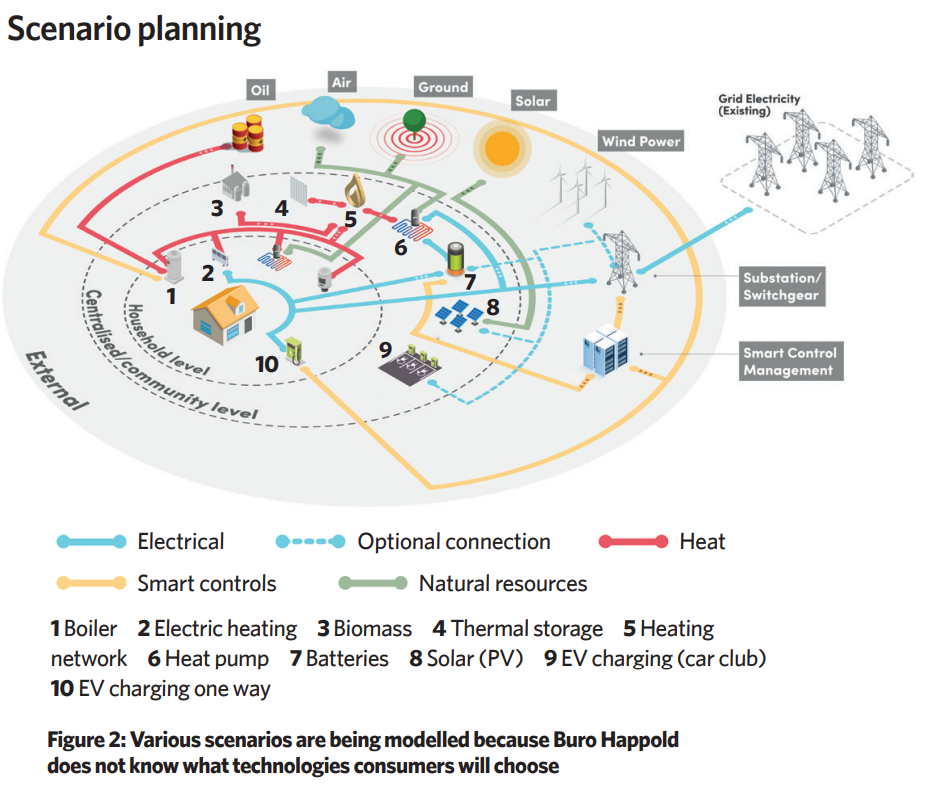
‘What we can say is that, if they made a certain choice, the model can anticipate what the outcome would be for consumers, in terms of their energy bill and capital cost, versus the implications for the network.’
In another scenario, the model will be used to assess the implications of adding a 7kW electric vehicle (EV) charger to the heating load for every home with a drive. Proctor explains: ‘The focus of CommuniHeat is about getting the heat price down and understanding the network impact of heat, but – from a network perspective – you also need to be able to understand the impact of combined heat and EV charging.’
The model will also be used to assess the impact on Grid demand of adding energy efficiency measures to homes. ‘We’ll model the impact of putting insulation into properties by adjusting the heat profile, which we’ll then convert into a power profile to assess the impact on the network,’ says Proctor
The team is now running the optimisation exercise, which will attempt to minimise the cost to the consumer of the oil to electric transition. This includes appraising the benefits of access to community energy services, which include community-owned renewable energy and new financing mechanisms. ‘Our results show community-owned assets and local energy markets can make a real difference to the cost to the consumer,’ says Proctor.
Other scenarios planned include modelling the impact of battery and thermal storage. ‘All these technologies are in the model. Once we’ve optimised consumer costs, we’ll look at demand flexibility,’ Proctor adds.
The resulting insights are being collated, and Proctor says these are proving really interesting and could lead to real changes in the way we approach decarbonisation for these communities.