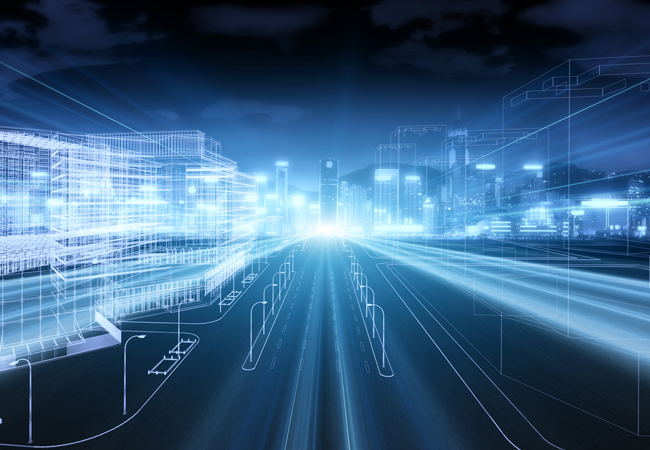
Figure 1: Deployment of a deep-learning model for real-time detection
Heating, ventilation and air conditioning (HVAC) systems and their associated operations are responsible for up to 40% of building energy consumption. Enhancing the efficiency or minimising the consumption of such systems will go a long way towards developing a low carbon economy and future. Solutions such as occupancy-based and demand-driven controls can achieve energy savings by eliminating unnecessary energy usage.
Occupants’ behaviour and thermal preferences can have a substantial impact on building energy consumption (Paone and Bacher, 2018). In conventional building management systems, HVAC networks operate on a fixed setpoint schedule, and assume maximum occupancy during working hours (Dong, 2018). These are typically based on load schedules predefined in standards such as ASHRAE 90.1 and Standard 55. Such solutions cannot reflect the stochastic and dynamic behaviour of occupancy patterns. The use of fixed setpoints in combination with varying occupancy could result in spaces being over- or under-conditioned.
This may lead to significant waste in energy consumption and affect occupancy thermal comfort and satisfaction (Kwok et al, 2011). This indicates the need for the development of solutions, such as demand driven controls, that adapt to occupancy patterns in real time and optimise HVAC operations, while providing comfortable conditions.
Compared with shallow-learning methods, deep-learning techniques can lead to better performance in terms of detection and recognition of various objects
Information on real-time occupancy patterns is central to the effective development and implementation of a demand-driven control strategy for HVAC. Conventional technologies – such as infrared, CO2 and temperature sensors – can be used to gather occupancy information by counting people in spaces. However, they may not give accurate, reliable data on the actual indoor environmental conditions (Tang et al, 2019). For example, they cannot sense the actual activities performed by occupants, which can affect indoor environment conditions.
Artificial intelligence (AI) techniques, such as deep learning, are becoming effective in improving HVAC system performances through building energy forecasting and management. Several works have already implemented vision-based deep-learning methods to identify human activities (Tien et al, 2020).
However, most of the work to date has attempted to improve the performance and accuracy of the deep-learning model for human presence detection and activity classification (Wei et al, 2019) rather than using the data to seek solutions for minimising building energy loads. Furthermore, no work has tried to predict the associated sensible and latent heat emission from the occupants, which affects the temperature and humidity levels in an internal space.
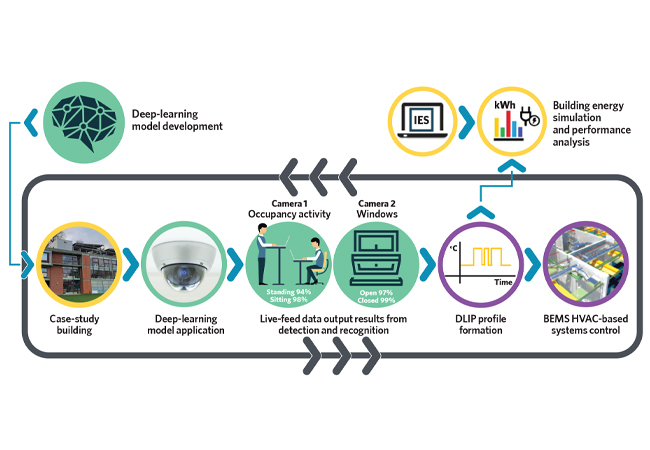
Figure 1: Deployment of a deep-learning model for real-time detection
The paper Energy management and optimisation of HVAC systems using a deep learning approach, presented at the 10th CIBSE ASHRAE Technical Symposium 2020, introduces a deep-learning-based framework for building energy management systems, to enable real-time detection and recognition of occupants’ activities within office building spaces.
The proposed approach is detailed in Figure 1. The first stage focuses on developing and deploying a deep learning model for real-time detection and recognition of occupancy behaviour within a building space. This is configured and trained using deep-learning techniques, and then validated before being used in an AI-powered camera. Compared with shallow-learning methods, deep learning techniques can lead to better performance in terms of detection and recognition of various objects.
Images of occupants’ activities – including napping, sitting, standing and walking – are collected, manually labelled, and used as a training dataset for the model. A second model is also developed, focusing on the detection and recognition of the opening and closing of windows by occupants. Specialist software was used for training the models.
An initial experiment was conducted within an office environment to test the capabilities and accuracy of the proposed approach. Results showed an average detection of 92% for occupancy activities and 78% for window status.
The second stage focuses on the formation of a profile, based on the detected and recognised occupancy number and activities; this is also called the deep-learning influenced profile (DLIP). This corresponds to each of the detected occupancy activities, which was coupled with the heat-emission rates of occupant-performing activities within an office (CIBSE Guide A). For the window detection model, the DLIP was based on the window conditions. Using collected information, the building energy management system can adjust the heating, ventilation and air conditioning systems automatically, to meet actual demands of the spaces in real time.
Figure 2 shows a process of DLIP formation for the live detection within a selected office space. The images are only for visualisation purposes. In practice, the current approach will output heat emission profiles, not actual occupancy information. To assess its feasibility and analyse its potential impact on building energy use, simulations were carried out to perform energy modelling of the case-study building.
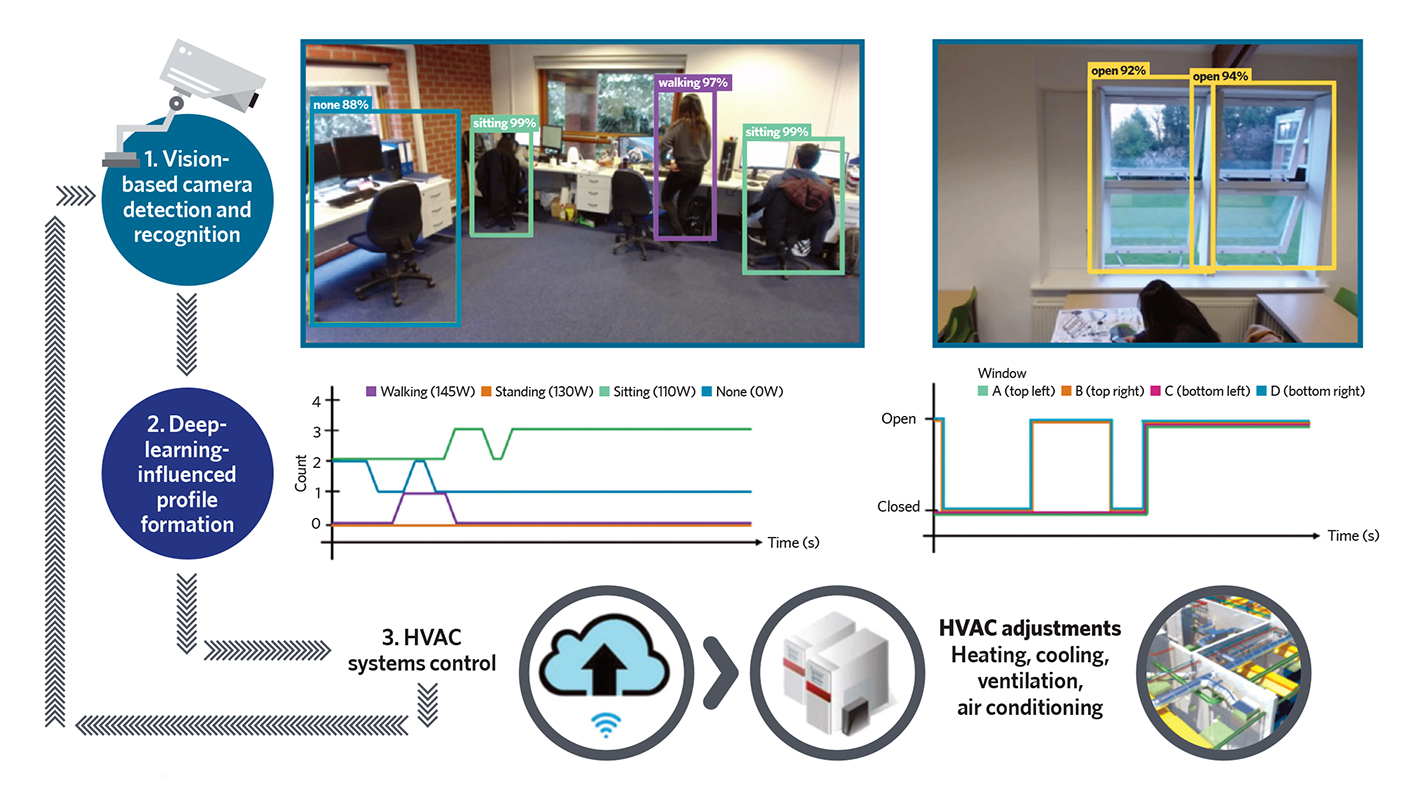
Process of deep learning and live detection in an office space
Based on initial application of the occupancy model within a standard office space, both the ‘static’ and DLIP were assigned. These were used to assess the potential energy savings that can be achieved. Results suggest use of the DLIP can prevent overestimation of occupancy heating gains by up to an average of 35%. Furthermore, the high detection accuracy resulted in an average difference of only 2.3% between DLIP results and the actual observation profile. Similarly, results achieved from the application of the window-detection model with building energy simulation suggests an effective solution for monitoring windows, especially when they are left open unintentionally.
Future research will study the system in other indoor environments and look at factors that have an effect on detection accuracy, such as the position of cameras and the room environmental conditions.
- The paper Energy management and optimisation of HVAC systems using a deep learning approach was voted most significant contribution to the art and science of building services engineering at the symposium.
- Paige Wenbin Tien is a PhD researcher in the Buildings, Energy and Environment Research Group at the Department of Architecture and Built Environment, University of Nottingham. The project is supported by the Engineering and Physical Sciences Research Council.